Programme
A detailed programme will follow but the general outline of the workshop is as follows:
- Tuesday 13th: Introduction to Machine Learning for data-intensive science
Time Session Speaker Slides 9:00-10:00 Coffee and Registration 10:00-10:05 Welcome & Logistics Alex Clarke Download 10:05-10:15 Workshop Overview & Open Science Rachael Ainsworth 10:15-10:30 Intro to JBCA Sara Summerton Download 10:30-11:00 Machine Learning Basics Alex Clarke Download 11:00-11:25 Introduction to Neural Networks Therese Cantwell Download 11:25-11:35 Comfort Break 11:35-12:05 Introduction to Support Vector Machines Joe Fennell Download 12:05-12:30 Introduction to Random Forests & t-SNE Alex Clarke Download 12:30-13:45 Lunch 13:45-14:30 Machine Learning with Music & Audio Alex Clarke Download 14:30-15:15 Machine Learning with Satellite data Joe Fennell Download 15:15-15:30 Comfort Break 15:30-16:15 Fine-Tuning Convolutional Neural Networks Therese Cantwell Download 16:15-19:00 Pizza social Lovell Room. Alan Turing Building - Wednesday 14th: Machine Learning applications and methods
Time Session Speaker Slides 8:45-9:15 Coffee and Registration 9:15-9:30 Day 2 Introduction Anna Scaife 9:30-10:15 The Automation of Science Ross King 10:15-11:00 Flash Talks 1 - Joe Hanson
- Richard Henchman
- Ann Gledson
- Matt Crooks
11:00-11:15 Comfort Break 11:15-12:00 Data-driven optimization - Designing meta-heuristics for data-rich optimization settings. Julia Handl 12:00-12:30 Flash Talks 2 - Karim Sidaoui
- Hongmin Tang
- Arianna Dagliati
12:30-13:45 Lunch 13:45-14:30 An overview of the ML and Data Science work at IBM research UK Blair Edwards 14:30-15:15 Assessing Mental Health through Social Media and Smart Devices: Current State, Challenges and Future Directions Adam Tsakalidis Download 15:15-15:30 Comfort Break 15:30-16:15 Learning at Scale Sidharth Kashyap 16:16-17:00 Flash Talks 3 - Stephanie Shoop-Worrall
- Zafiirah Hosenie
- Alexia Sampri
17:00-18:00 Drinks Reception - Thursday 15th: Machine Learning applications and methods
Time Session Speaker Slides 8:30-9:00 Coffee 9:00-9:45 Toward learning from few examples ('one-shot' learning) using neuromorphic hardware Kamelia Demova 9:45-10:30 Online Inference Using Distribution-Valued Stochastic Processes Thomas House 10:30-10:45 Comfort Break 10:45-11:30 Shipping a Machine Learning model to Production; is it always smooth sailing? Leanne Fitzpatrick Download 11:30-12:14 Flash Talks 4 - Georgia Ionescu
12:15-12:45 Discussion and closing comments 12:45-13:45 Lunch
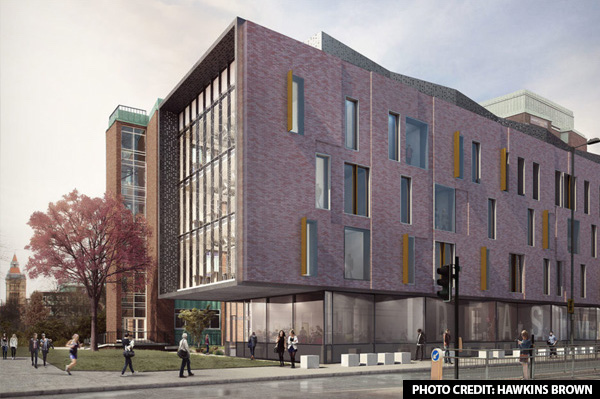
Venue
The workshop will take place in the Bell-Burnell Lecture Room located in the Schuster Building on the University of Manchester's main campus.
Invited Speakers
We have invited speakers from both academia and industry to talk about the latest research and applications in Machine Learning:
Flash Talk Abstracts
There will be a number of flash talk on Wednesday and Thursday:
Name | Abstract | Flash Talk |
---|---|---|
Joe Hanson | I'm applying machine learning to research cosmic magnetism in astrophysics, and my work involves classification with convolutional neural networks. This novel application of machine learning also necessitates further research into some specific problems; including handling class imbalance, and transitioning (e.g. through transfer or semi-supervised learning) from labelled simulated datasets to real (and unlabelled) observations. | Flash Talks 1 |
Richard Henchman | We develop theory for the total quantification of molecular flexibility using computer simulation. A key challenge is to automate the process so that it works for any simulation. | Flash Talks 1 |
Ann Gledson | Ann has been conducting research since 2000 in the areas of data analytics, text mining, interface design and software engineering and she will give an overview of the variety of data science tasks she has recently worked on in her current role as a Research Software Engineer at the UoM. For example in the InnovateUK CityVerve project she developed a web application to combine disparate streams of IoT data and allows users to perform various analysis techniques. | Flash Talks 1 |
Matt Crooks | How well can we differentiate school days from school holidays using viewing figures of CBBC programmes on BBC iPlayer? In fact, well enough to identify snow days! | Flash Talks 1 |
Karim Sidaoui | Understanding customer experience using artificial intelligence and machine learning. | Flash Talks 2 |
Hongmin Tang | Classifying radio galaxy morphology has been done by visual inspection in the last few decades. Given that CNN can extract features from image data, we adopted a 13-layer convolutional network architecture to classify selected radio survey images. The best approach yielded over 90% of general model accuracy. Some transfer learning approach were done as well. | Flash Talks 2 |
Arianna Dagliati | Use of Topological Data Analysis approaches to tackle precision medicine issues. Application of unsupervised methods to identify temporal phenotypes. | Flash Talks 2 |
Stephanie Shoop-Worrall | There are six key outcomes for young people with arthritis which may change over time in different directions. We aim to understand if groups of young people experience different outcome patterns. | Flash Talks 3 |
Zafiirah Hosenie | I will outline the application of machine learning techniques for variable star classification. In particular, I will demonstrate various methods for feature visualisation and evaluation applicable to high dimensional datasets. | Flash Talks 3 |
Alexia Sampri | Challenges in the aggregation of biomedical datasets and probabilistic approaches to overcome representational heterogeneity - Systemic Lupus Erythematosus exemplar | Flash Talks 3 |
Georgia Ionescu | Deep learning can be used for predicting breast cancer by analysing mammographic images. Our method has been tested on a large dataset and showed results comparable with human readers (medical experts). | Flash Talks 4 |
Register
Registration is now closed.